Open Problems in Single-Cell Analysis
Single-cell analysis is hard. Open Problems aims to define and assess those challenges.
Benchmarking formalized challenges in single-cell analysis
Advances in microfluidic technology have transformed single-cell analysis, allowing for unprecedented data collection. However, these complex datasets require more than standard statistical techniques.
To fully harness the potential of single-cell biology, we need new methods of data analysis. Our goal is to formalize key challenges and create a community-driven platform to benchmark and advance these methods.
Our inspiration
Learning from machine learning
We are inspired by breakthroughs in machine learning, driven by competitions like ImageNet (computer vision), the Workshop on Statistical Machine Translation (NLP), and the Netflix Prize (recommendations), which have collectively pushed the boundaries of what AI systems can achieve in various domains.
Formalized challenges
Major advances in biological sciences, such as DeepMind's protein folding success with CASP and innovations from the Dream Challenges and RxRx competitions, highlight the impact of structured challenges.
Cross-disciplinary innovation
Computational single-cell methods have progressed, often leveraging advances from fields like computer vision. We aim to enhance single-cell analysis by fostering collaboration between machine learning and biomedical research.
Our approach
We believe four key traits drive innovation in challenges
Clear definitions
Tasks are mathematically well-defined.
Standardized datasets
Public, ready-to-use gold-standard datasets.
Quantitative metrics
Success is measured by clear metrics.
Continuous leaderboards
State-of-the-art methods are ranked and updated regularly.
Our sponsors
These organizations have contributed financially to Open Problems.
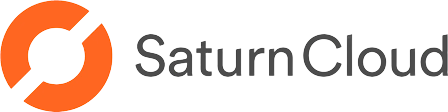
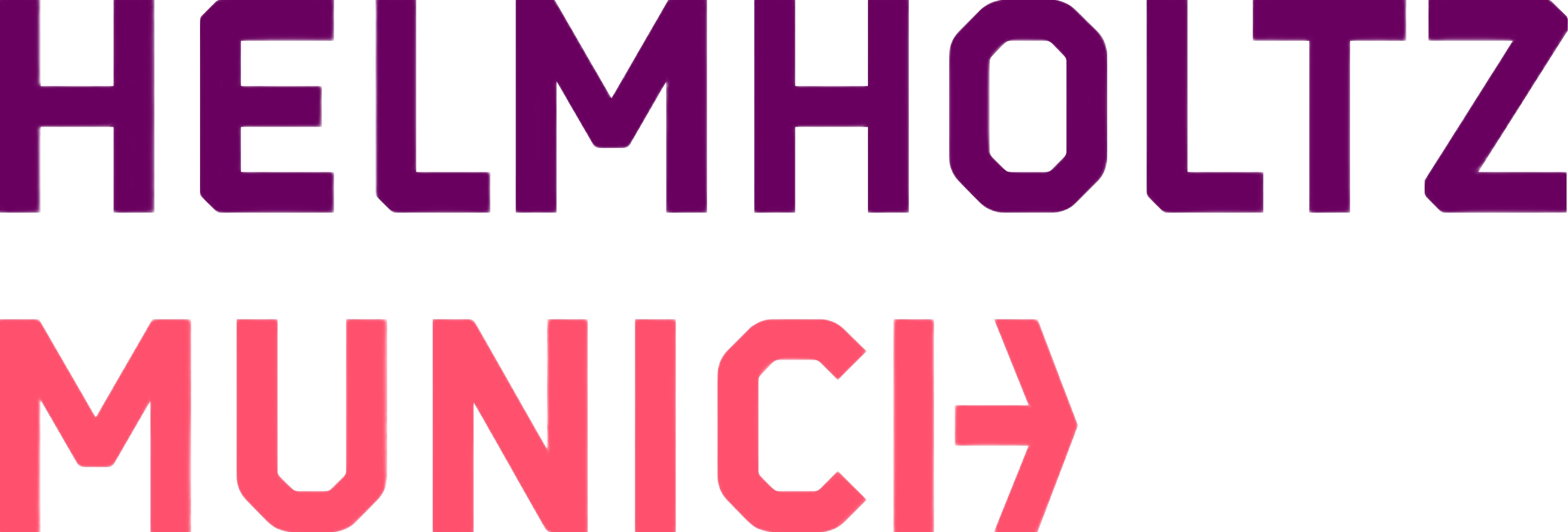
Who are we?
We are machine learning scientists, computational biologists, and single-cell data analysts who formalize computational tasks in single-cell analysis and collaborate with molecular biologists to generate benchmarking datasets that challenge methods and method developers to perform ever better.
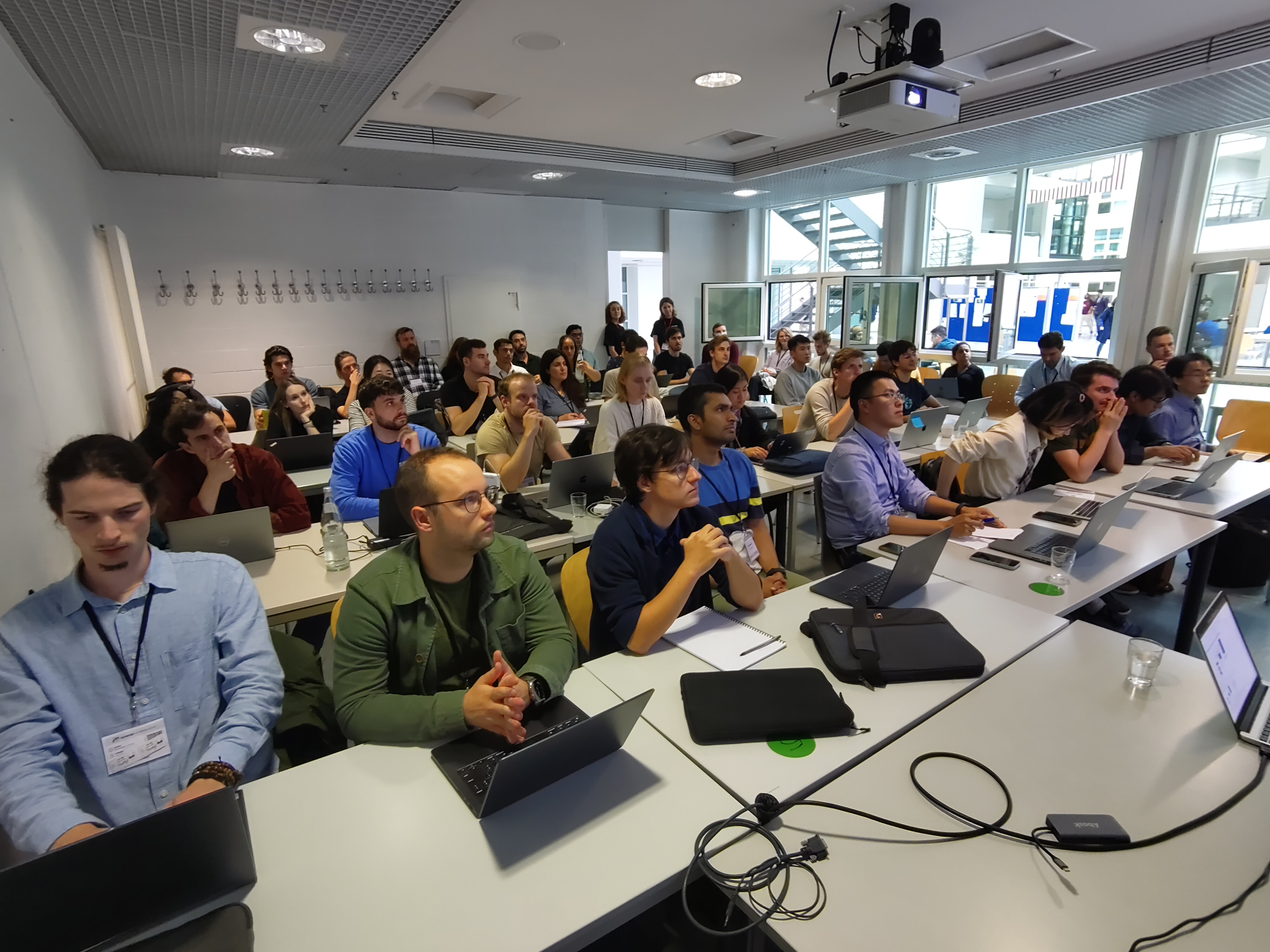
Join us!
We’d love for you to get involved.
You can start by joining our mailing list to be the first to hear about updates.
Next, check out our Contributing Guidelines.
Finally, introduce yourself by giving us a 👋 on our Discord Server! You’ll find several groups of people here working on different tasks. Check out the different channels and see where you can contribute!